
You might not have seen seq_along() before. It’s useful to think of i as a pronoun, like “it”. This determines what to loop over:Įach run of the for loop will assign i to a different value from “integer”, “double”, “character”, etc) and the length of the vector. It has two arguments: the type of the vector (“logical”, The for loop at each iteration using c() (for example), your for loopĪ general way of creating an empty vector of given length is the vector()įunction. This is very important for efficiency: if you grow The output: output <- vector("double", length(x)).īefore you start the loop, you must always allocate sufficient spaceįor the output. Once you master the vocabulary of FP, you can solve many common iteration problems with less code, more ease, and fewer errors. Functional programming (FP) offers tools to extract out this duplicated code, so each common for loop pattern gets its own function.
#For if loop in r code
However, for loops are quite verbose, and require quite a bit of bookkeeping code that is duplicated for every for loop. On the imperative side you have tools like for loops and while loops, which are a great place to start because they make iteration very explicit, so it’s obvious what’s happening. In this chapter you’ll learn about two important iteration paradigms: imperative programming and functional programming. Another tool for reducing duplication is iteration, which helps you when you need to do the same thing to multiple inputs: repeating the same operation on different columns, or on different datasets. One tool for reducing duplication is functions, which reduce duplication by identifying repeated patterns of code and extract them out into independent pieces that can be easily reused and updated. You’re likely to have fewer bugs because each line of code is Remembering to change every place that you copied-and-pasted the As your needsĬhange, you only need to make changes in one place, rather than It’s easier to respond to changes in requirements.
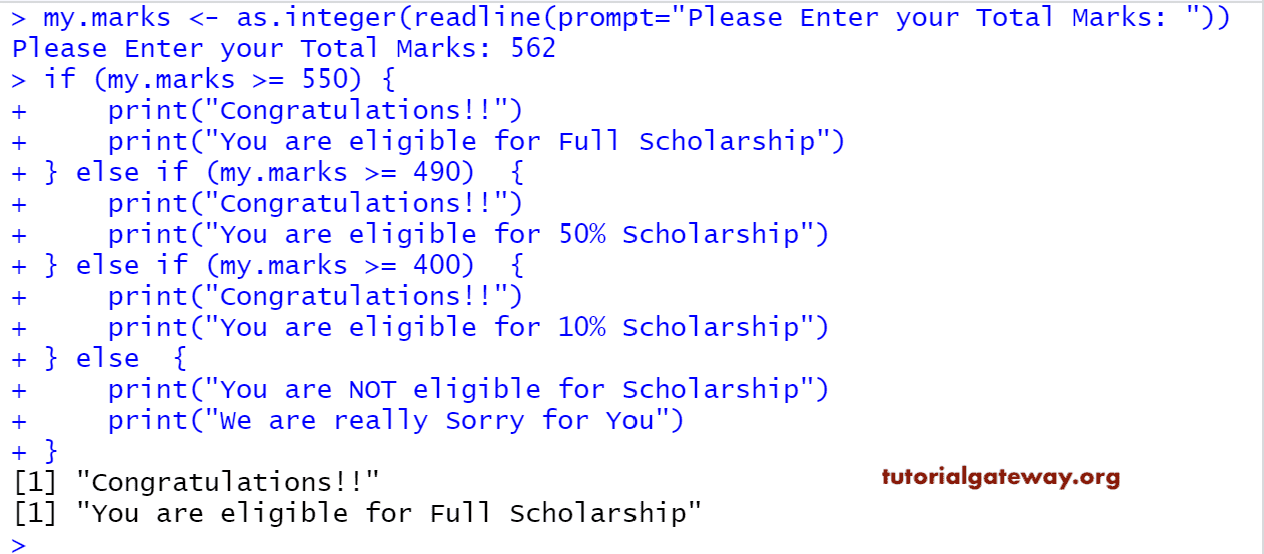
It’s easier to see the intent of your code, because your eyes areĭrawn to what’s different, not what stays the same. Reducing code duplication has three main benefits: How do we write a function? All functions in R have two parts: The input arguments and the body.In functions, we talked about how important it is to reduce duplication in your code by creating functions instead of copying-and-pasting. We may want to put this in a function so that we don’t have to worry about typing the number multiple times and ending up with typos like we did above. In this example, we have to multiply two different columns by a very long number and then add 10. Many functions you would commonly use are built, but you can create custom functions to do anything you want. When you take an average mean(), find the dimensions of something dim, or anything else where you type a command followed immediately by paratheses you are calling a function. Typos like these can happen anytime, and best practice is if you’re going to need to do something more than once, put it what’s called a function. Surveys_adjusted$hindfoot_length <- surveys$hindfoot_length* 1.1245697375093747 +10ĭo you see the problem above? While typing in that really long number, I accidently hit a 9 instead of an 8. Let’s save our adjusted data to our data folder:
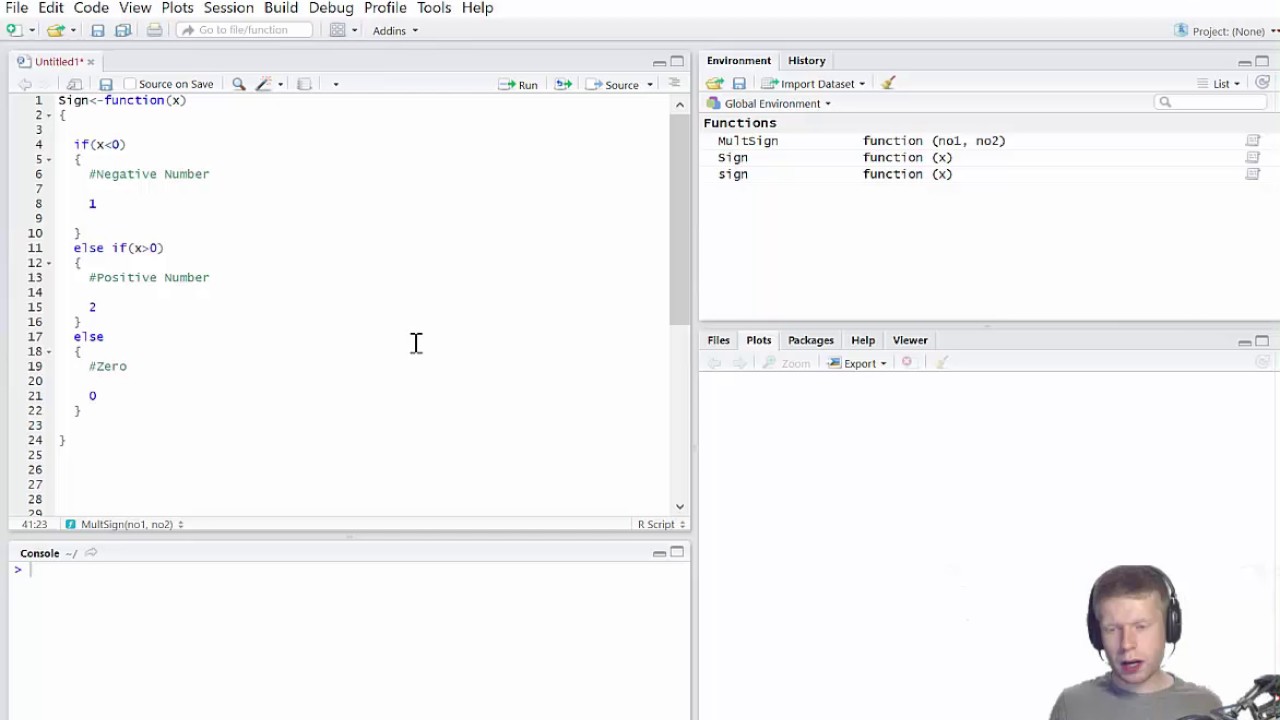
Putting quotes around each cell is the default and can be beneficial if you have special characters or a lot of spaces and tabs within a cell, however, most of the time you will not need this and should set quote=FALSE, especially if you plan on opening the saved file in a program other than R. The other three arguments above give instructions about whether you’d like to include the row names of the data, the column names of the data, and whether you’d like quotes to be put around each cell. You could also put sep="\t" for a tab-delimited file or sep="\n" if you want each cell to be in it’s own row.
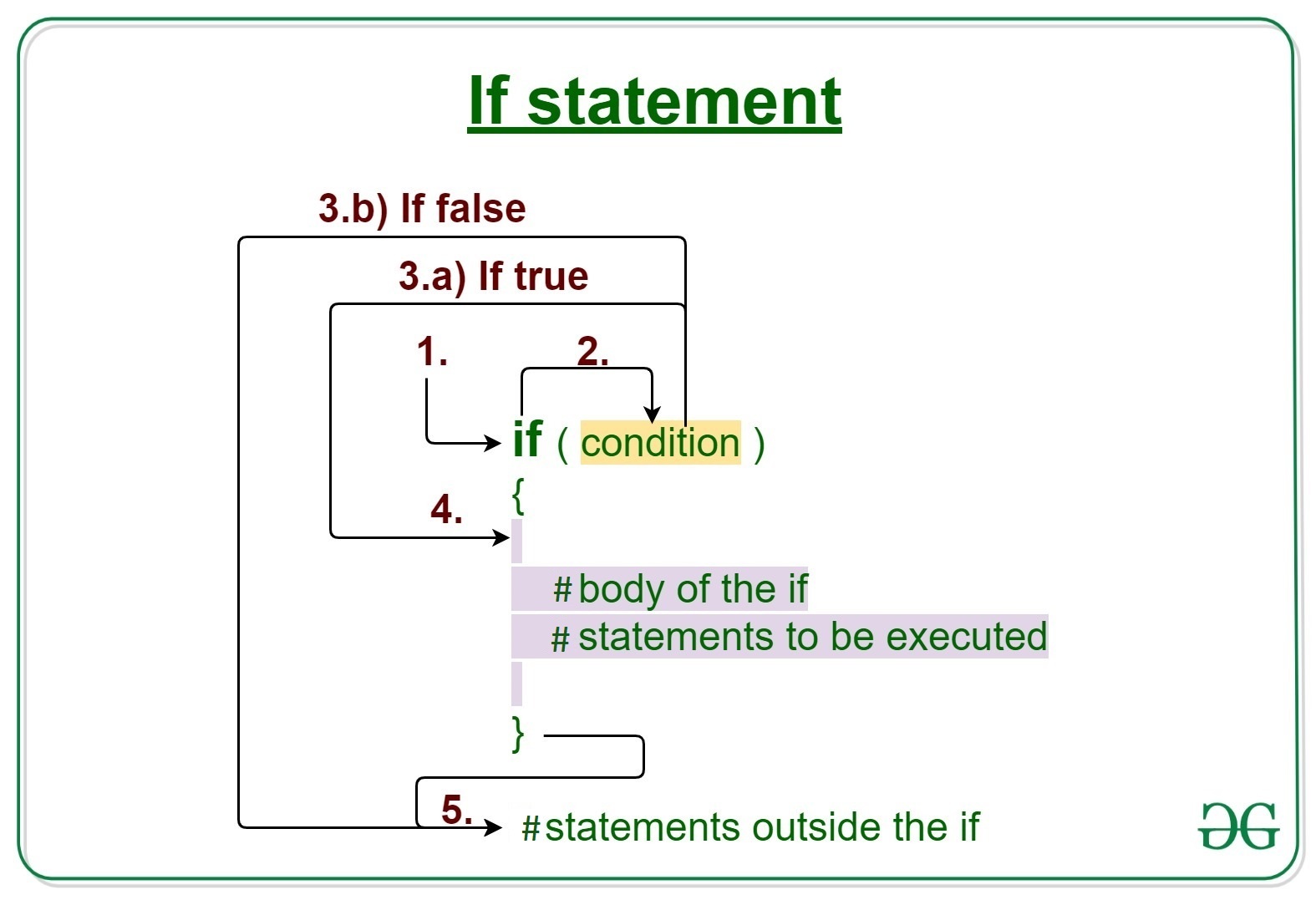
Here, we’ve put a, so this will create a. The sep arguement let’s you choose how you want the cells in your file to be delimited. Then you give it the path and name of file you want to save it to. The first arguement asks for the variable the table you wish to write out is stored. Write.table(table_variable, "name_of_file_to_write_to", sep= ",", row.names= FALSE, col.names= FALSE, quote= FALSE)
